How do AI picture turbines image the previous? New analysis signifies that they drop smartphones into the 18th century, insert laptops into Thirties scenes, and place vacuum cleaners in Nineteenth-century houses, elevating questions on how these fashions think about historical past – and whether or not they’re able to contextual historic accuracy in any respect.
Early in 2024, the image-generation capabilities of Google’s Gemini multimodal AI mannequin got here beneath criticism for imposing demographic equity in inappropriate contexts, akin to producing WWII German troopers with unlikely provenance:
Demographically inconceivable German navy personnel, as envisaged by Google’s Gemini multimodal mannequin in 2024. Supply: Gemini AI/Google through The Guardian
This was an instance the place efforts to redress bias in AI fashions did not take account of a historic context. On this case, the difficulty was addressed shortly after. Nonetheless, diffusion-based fashions stay liable to generate variations of historical past that confound trendy and historic points and artefacts.
That is partly due to entanglement, the place qualities that continuously seem collectively in coaching knowledge grow to be fused within the mannequin’s output. For instance, if trendy objects like smartphones typically co-occur with the act of speaking or listening within the dataset, the mannequin could study to affiliate these actions with trendy units, even when the immediate specifies a historic setting. As soon as these associations are embedded within the mannequin’s inner representations, it turns into troublesome to separate the exercise from its modern context, resulting in traditionally inaccurate outcomes.
A brand new paper from Switzerland, analyzing the phenomenon of entangled historic generations in latent diffusion fashions, observes that AI frameworks which can be fairly able to creating photorealistic folks nonetheless favor to depict historic figures in historic methods:
![From the new paper, diverse representations via LDM of the prompt' 'A photorealistic image of a person laughing with a friend in [the historical period]', with each period indicated in each output. As we can see, the medium of the era has become associated with the content. Source: https://arxiv.org/pdf/2505.17064](https://www.unite.ai/wp-content/uploads/2025/05/laughing-with-a-friend.jpg)
From the brand new paper, various representations through LDM of the immediate’ ‘A photorealistic picture of an individual laughing with a good friend in [the historical period]’, with every interval indicated in every output. As we are able to see, the medium of the period has grow to be related to the content material. Supply: https://arxiv.org/pdf/2505.17064
For the immediate ‘A photorealistic picture of an individual laughing with a good friend in [the historical period]’, one of many three examined fashions typically ignores the adverse immediate ‘monochrome’ and as an alternative makes use of coloration therapies that mirror the visible media of the required period, as an example mimicking the muted tones of celluloid movie from the Nineteen Fifties and Nineteen Seventies.
In testing the three fashions for his or her capability to create anachronisms (issues which aren’t of the goal interval, or ‘out of time’ – which can be from the goal interval’s future in addition to its previous), they discovered a common disposition to conflate timeless actions (akin to ‘singing’ or ‘cooking’) with trendy contexts and tools:
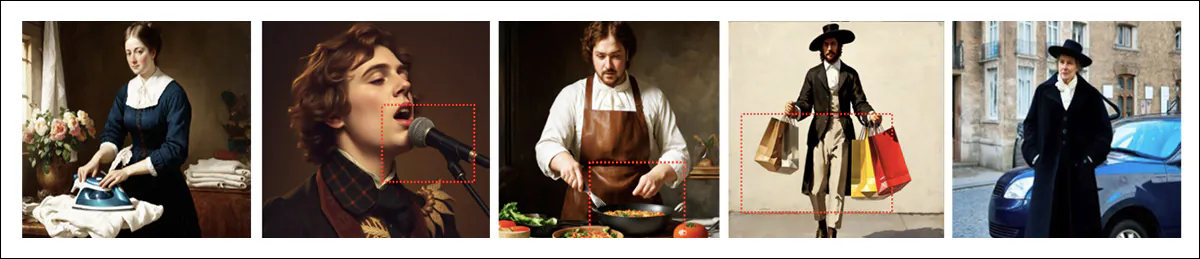
Numerous actions which can be completely legitimate for earlier centuries are depicted with present or more moderen know-how and paraphernalia, in opposition to the spirit of the requested imagery.
Of notice is that smartphones are significantly troublesome to separate from the idiom of images, and from many different historic contexts, since their proliferation and depiction is well-represented in influential hyperscale datasets akin to Widespread Crawl:
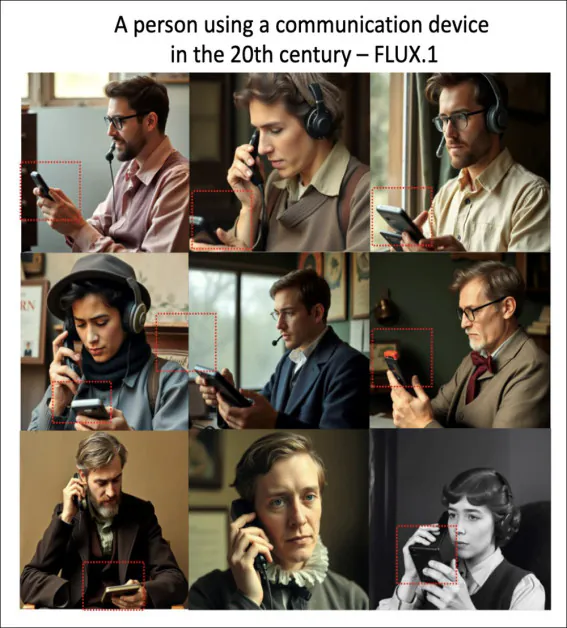
Within the Flux generative text-to-image mannequin, communications and smartphones are tightly-associated ideas – even when historic context doesn’t allow it.
To find out the extent of the issue, and to provide future analysis efforts a method ahead with this explicit bugbear, the brand new paper’s authors developed a bespoke dataset in opposition to which to check generative techniques. In a second, we’ll check out this new work, which is titled Artificial Historical past: Evaluating Visible Representations of the Previous in Diffusion Fashions, and comes from two researchers on the College of Zurich. The dataset and code are publicly out there.
A Fragile ‘Fact’
A few of the themes within the paper contact on culturally delicate points, such because the under-representation of races and gender in historic representations. Whereas Gemini’s imposition of racial equality within the grossly inequitable Third Reich is an absurd and insulting historic revision, restoring ‘conventional’ racial representations (the place diffusion fashions have ‘up to date’ these) would typically successfully ‘re-whitewash’ historical past.
Many latest hit historic reveals, akin to Bridgerton, blur historic demographic accuracy in methods more likely to affect future coaching datasets, complicating efforts to align LLM-generated interval imagery with conventional requirements. Nonetheless, this can be a advanced matter, given the historic tendency of (western) historical past to favor wealth and whiteness, and to go away so many ‘lesser’ tales untold.
Making an allowance for these difficult and ever-shifting cultural parameters, let’s check out the researchers’ new method.
Technique and Exams
To check how generative fashions interpret historic context, the authors created HistVis, a dataset of 30,000 pictures produced from 100 prompts depicting frequent human actions, every rendered throughout ten distinct time intervals:
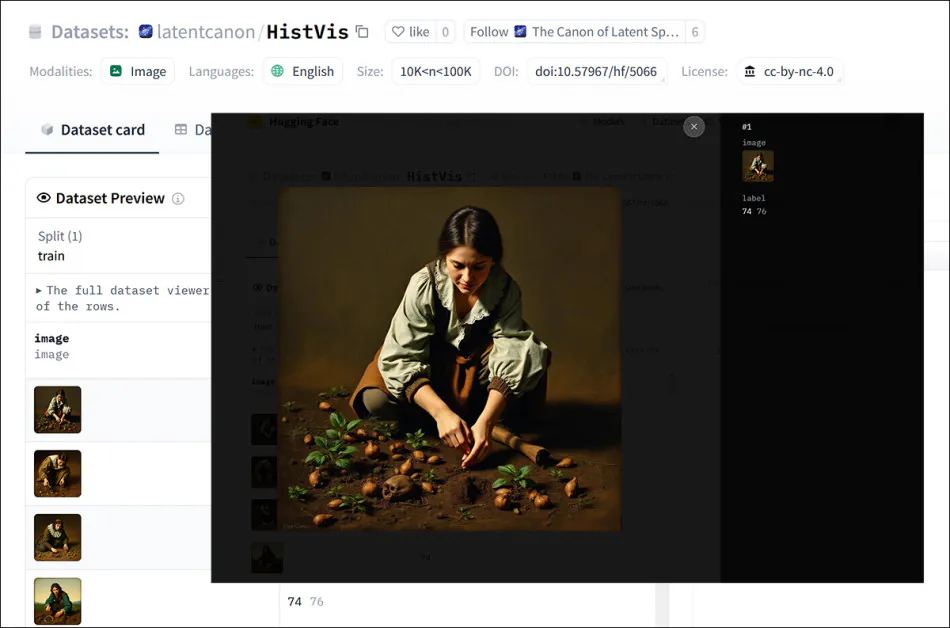
A pattern from the HistVis dataset, which the authors have made out there at Hugging Face. Supply: https://huggingface.co/datasets/latentcanon/HistVis
The actions, akin to cooking, praying or listening to music, had been chosen for his or her universality, and phrased in a impartial format to keep away from anchoring the mannequin in any explicit aesthetic. Time intervals for the dataset vary from the seventeenth century to the current day, with added give attention to 5 particular person many years from the 20th century.
30,000 pictures had been generated utilizing three widely-used open-source diffusion fashions: Steady Diffusion XL; Steady Diffusion 3; and FLUX.1. By isolating the time interval as the one variable, the researchers created a structured foundation for evaluating how historic cues are visually encoded or ignored by these techniques.
Visible Fashion Dominance
The writer initially examined whether or not generative fashions default to particular visible types when depicting historic intervals; as a result of it appeared that even when prompts included no point out of medium or aesthetic, the fashions would typically affiliate explicit centuries with attribute types:
![Predicted visual styles for images generated from the prompt “A person dancing with another in the [historical period]” (left) and from the modified prompt “A photorealistic image of a person dancing with another in the [historical period]” with “monochrome picture” set as a negative prompt (right).](https://www.unite.ai/wp-content/uploads/2025/05/period-style.jpg)
Predicted visible types for pictures generated from the immediate ‘An individual dancing with one other within the [historical period]’ (left) and from the modified immediate ‘A photorealistic picture of an individual dancing with one other within the [historical period]’ with ‘monochrome image’ set as a adverse immediate (proper).
To measure this tendency, the authors educated a convolutional neural community (CNN) to categorise every picture within the HistVis dataset into certainly one of 5 classes: drawing; engraving; illustration; portray; or images. These classes had been supposed to mirror frequent patterns that emerge throughout time-periods, and which assist structured comparability.
The classifier was based mostly on a VGG16 mannequin pre-trained on ImageNet and fine-tuned with 1,500 examples per class from a WikiArt-derived dataset. Since WikiArt doesn’t distinguish monochrome from coloration images, a separate colorfulness rating was used to label low-saturation pictures as monochrome.
The educated classifier was then utilized to the complete dataset, with the outcomes displaying that each one three fashions impose constant stylistic defaults by interval: SDXL associates the seventeenth and 18th centuries with engravings, whereas SD3 and FLUX.1 have a tendency towards work. In twentieth-century many years, SD3 favors monochrome images, whereas SDXL typically returns trendy illustrations.
These preferences had been discovered to persist regardless of immediate changes, suggesting that the fashions encode entrenched hyperlinks between fashion and historic context.
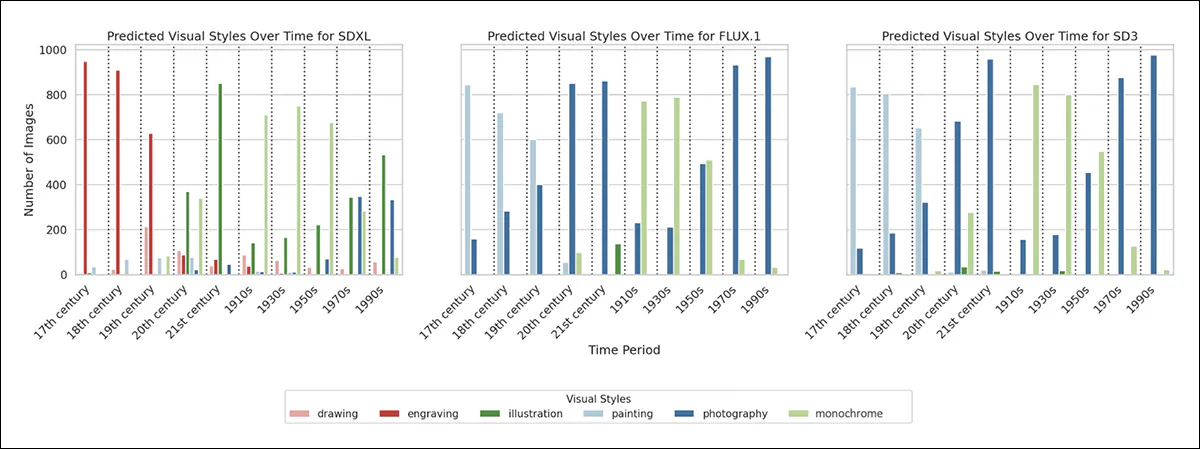
Predicted visible types of generated pictures throughout historic intervals for every diffusion mannequin, based mostly on 1,000 samples per interval per mannequin.
To quantify how strongly a mannequin hyperlinks a historic interval to a selected visible fashion, the authors developed a metric they title Visible Fashion Dominance (VSD). For every mannequin and time interval, VSD is outlined because the proportion of outputs predicted to share the commonest fashion:
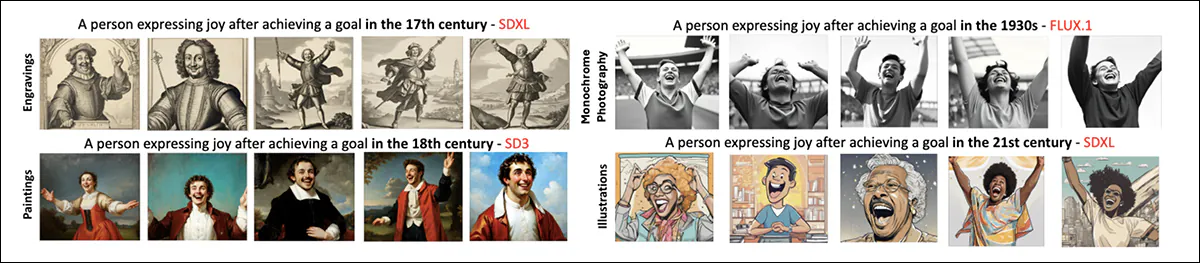
Examples of stylistic biases throughout the fashions.
The next rating signifies {that a} single fashion dominates the outputs for that interval, whereas a decrease rating factors to better variation. This makes it doable to match how tightly every mannequin adheres to particular stylistic conventions throughout time.
Utilized to the complete HistVis dataset, the VSD metric reveals differing ranges of convergence, serving to to make clear how strongly every mannequin narrows its visible interpretation of the previous:
The outcomes desk above reveals VSD scores throughout historic intervals for every mannequin. Within the seventeenth and 18th centuries, SDXL tends to provide engravings with excessive consistency, whereas SD3 and FLUX.1 favor portray. By the twentieth and twenty first centuries, SD3 and FLUX.1 shift towards images, whereas SDXL reveals extra variation, however typically defaults to illustration.
All three fashions show a robust choice for monochrome imagery in earlier many years of the twentieth century, significantly the 1910s, Thirties and Nineteen Fifties.
To check whether or not these patterns may very well be mitigated, the authors used immediate engineering, explicitly requesting photorealism and discouraging monochrome output utilizing a adverse immediate. In some circumstances, dominance scores decreased, and the main fashion shifted, as an example, from monochrome to portray, within the seventeenth and 18th centuries.
Nonetheless, these interventions not often produced genuinely photorealistic pictures, indicating that the fashions’ stylistic defaults are deeply embedded.
Historic Consistency
The subsequent line of study checked out historic consistency: whether or not generated pictures included objects that didn’t match the time interval. As a substitute of utilizing a set listing of banned gadgets, the authors developed a versatile methodology that leveraged massive language (LLMs) and vision-language fashions (VLMs) to identify parts that appeared misplaced, based mostly on the historic context.
The detection methodology adopted the identical format because the HistVis dataset, the place every immediate mixed a historic interval with a human exercise. For every immediate, GPT-4o generated a listing of objects that will be misplaced within the specified time interval; and for each proposed object, GPT-4o produced a yes-or-no query designed to verify whether or not that object appeared within the generated picture.
For instance, given the immediate ‘An individual listening to music within the 18th century’, GPT-4o would possibly determine trendy audio units as traditionally inaccurate, and produce the query Is the particular person utilizing headphones or a smartphone that didn’t exist within the 18th century?.
These questions had been handed again to GPT-4o in a visible question-answering setup, the place the mannequin reviewed the picture and returned a sure or no reply for every. This pipeline enabled detection of traditionally implausible content material with out counting on any predefined taxonomy of recent objects:
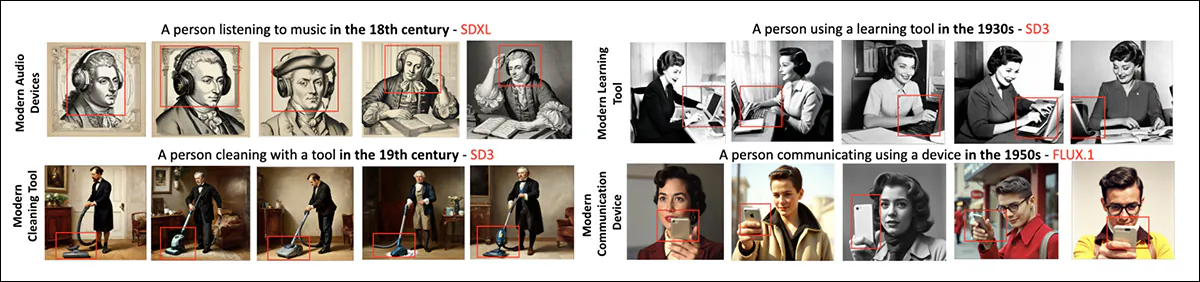
Examples of generated pictures flagged by the two-stage detection methodology, displaying anachronistic parts: headphones within the 18th century; a vacuum cleaner within the Nineteenth century; a laptop computer within the Thirties; and a smartphone within the Nineteen Fifties.
To measure how typically anachronisms appeared within the generated pictures, the authors launched a easy methodology for scoring frequency and severity. First, they accounted for minor wording variations in how GPT-4o described the identical object.
For instance, trendy audio system and digital audio system had been handled as equal. To keep away from double-counting, a fuzzy matching system was used to group these surface-level variations with out affecting genuinely distinct ideas.
As soon as all proposed anachronisms had been normalized, two metrics had been computed: frequency measured how typically a given object appeared in pictures for a particular time interval and mannequin; and severity measured how reliably that object appeared as soon as it had been instructed by the mannequin.
If a contemporary telephone was flagged ten occasions and appeared in ten generated pictures, it acquired a severity rating of 1.0. If it appeared in solely 5, the severity rating was 0.5. These scores helped determine not simply whether or not anachronisms occurred, however how firmly they had been embedded within the mannequin’s output for every interval:
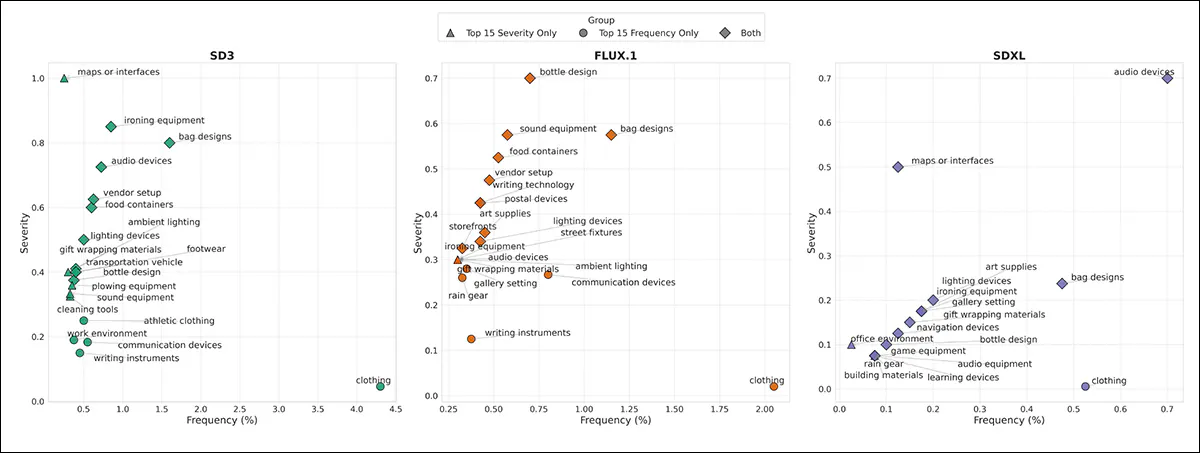
High fifteen anachronistic parts for every mannequin, plotted by frequency on the x-axis and severity on the y-axis. Circles mark parts ranked within the high fifteen by frequency, triangles by severity, and diamonds by each.
Above we see the fifteen most typical anachronisms for every mannequin, ranked by how typically they appeared and the way constantly they matched prompts.
Clothes was frequent however scattered, whereas gadgets like audio units and ironing tools appeared much less typically, however with excessive consistency – patterns that recommend the fashions typically reply to the exercise within the immediate greater than the time interval.
SD3 confirmed the very best price of anachronisms, particularly in Nineteenth-century and Thirties pictures, adopted by FLUX.1 and SDXL.
To check how nicely the detection methodology matched human judgment, the authors ran a user-study that includes 1,800 randomly-sampled pictures from SD3 (the mannequin with the very best anachronism price), with every picture rated by three crowd-workers. After filtering for dependable responses, 2,040 judgments from 234 customers had been included, and the strategy agreed with the bulk vote in 72 % of circumstances.
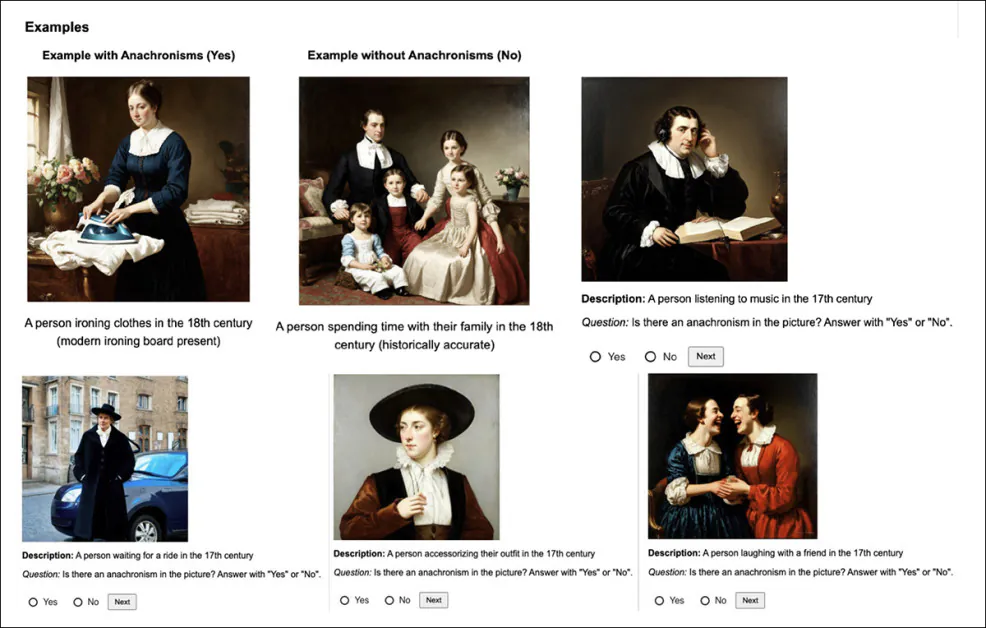
GUI for the human analysis examine, displaying activity directions, examples of correct and anachronistic pictures, and yes-no questions for figuring out temporal inconsistencies in generated outputs.
Demographics
The ultimate evaluation checked out how fashions painting race and gender over time. Utilizing the HistVis dataset, the authors in contrast mannequin outputs to baseline estimates generated by a language mannequin. These estimates weren’t exact however provided a tough sense of historic plausibility, serving to to disclose whether or not the fashions tailored depictions to the supposed interval.
To evaluate these depictions at scale, the authors constructed a pipeline evaluating model-generated demographics to tough expectations for every time and exercise. They first used the FairFace classifier, a ResNet34-based software educated on over 100 thousand pictures, to detect gender and race within the generated outputs, permitting for measurement of how typically faces in every scene had been categorized as male or feminine, and for the monitoring of racial classes throughout intervals.
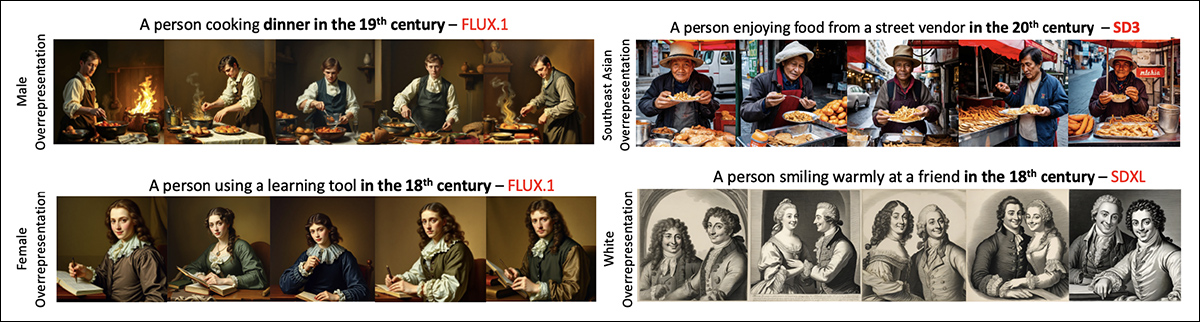
Examples of generated pictures displaying demographic overrepresentation throughout totally different fashions, time intervals and actions.
Low-confidence outcomes had been filtered out to cut back noise, and predictions had been averaged over all pictures tied to a particular time and exercise. To verify the reliability of the FairFace readings, a second system based mostly on DeepFace was used on a pattern of 5,000 pictures. The 2 classifiers confirmed sturdy settlement, supporting the consistency of the demographic readings used within the examine.
To check mannequin outputs with historic plausibility, the authors requested GPT-4o to estimate the anticipated gender and race distribution for every exercise and time interval. These estimates served as tough baselines quite than floor reality. Two metrics had been then used: underrepresentation and overrepresentation, measuring how a lot the mannequin’s outputs deviated from the LLM’s expectations.
The outcomes confirmed clear patterns: FLUX.1 typically overrepresented males, even in eventualities akin to cooking, the place ladies had been anticipated; SD3 and SDXL confirmed comparable developments throughout classes akin to work, training and faith; white faces appeared greater than anticipated total, although this bias declined in more moderen intervals; and a few classes confirmed surprising spikes in non-white illustration, suggesting that mannequin habits could mirror dataset correlations quite than historic context:
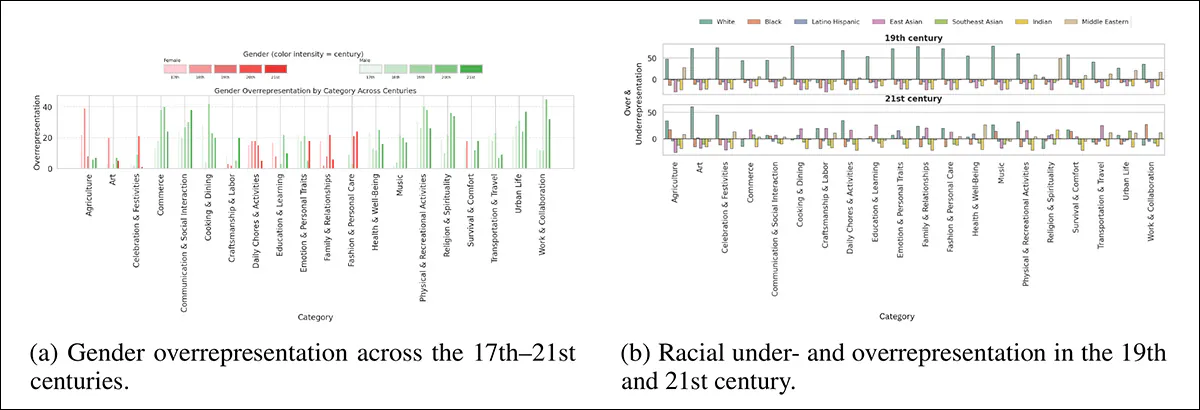
Gender and racial overrepresentation and underrepresentation in FLUX.1 outputs throughout centuries and actions, proven as absolute variations from GPT-4o demographic estimates.
The authors conclude:
‘Our evaluation reveals that [Text-to-image/TTI] fashions depend on restricted stylistic encodings quite than nuanced understandings of historic intervals. Every period is strongly tied to a particular visible fashion, leading to one-dimensional portrayals of historical past.
‘Notably, photorealistic depictions of individuals seem solely from the twentieth century onward, with solely uncommon exceptions in FLUX.1 and SD3, suggesting that fashions reinforce realized associations quite than flexibly adapting to historic contexts, perpetuating the notion that realism is a contemporary trait.
‘As well as, frequent anachronisms recommend that historic intervals will not be cleanly separated within the latent areas of those fashions, since trendy artifacts typically emerge in pre-modern settings, undermining the reliability of TTI techniques in training and cultural heritage contexts.’
Conclusion
Throughout the coaching of a diffusion mannequin, new ideas don’t neatly settle into predefined slots throughout the latent house. As a substitute, they kind clusters formed by how typically they seem and by their proximity to associated concepts. The result’s a loosely-organized construction the place ideas exist in relation to their frequency and typical context, quite than by any clear or empirical separation.
This makes it troublesome to isolate what counts as ‘historic’ inside a big, general-purpose dataset. Because the findings within the new paper recommend, many time intervals are represented extra by the look of the media used to depict them than by any deeper historic element.
That is one purpose it stays troublesome to generate a 2025-quality photorealistic picture of a personality from (as an example) the Nineteenth century; most often, the mannequin will depend on visible tropes drawn from movie and tv. When these fail to match the request, there may be little else within the knowledge to compensate. Bridging this hole will seemingly depend upon future enhancements in disentangling overlapping ideas.
First revealed Monday, Could 26, 2025